It has been an exciting collaboration between a range of research groups from different backgrounds to design ImmunoCluster, which will allow a far wider range of labs to apply unsupervised computational approaches to analysing their cytometry data.
Dr James Arnold, School of Cancer & Pharmaceutical Sciences
14 June 2021
New open-source easy-to-use resource for the analysis of high-dimensional cytometry data launches
ImmunoCluster provides a computational framework for the non-specialist to profile complex high-dimensional data such as mass and flow cytometry.

Dr Shahram Kordasti and Dr James Arnold both lead research groups within the School of Cancer & Pharmaceutical Sciences, both of which are focused on cancer immunology. A collaborative effort between the groups, as well as other international collaborators, has seen the development of ImmunoCluster, an open-source computational package that allows users to analyse high-dimensional cytometry data which has just been published in eLife.
High-dimensional cytometry approaches, which allow a researcher to study a cell’s characteristics, are essential approaches for dissecting the immune response in both healthy and diseased individuals and can provide novel insight into the underlying biology of a disease and identify biomarkers that may aid patient stratification or predict patient outcome.
The literature is wealthy with different packages to analyse high-dimensional data, but we wanted to design a computational framework that would incorporate all state-of-the-art packages for the analysis of liquid and imaging mass cytometry, and flow cytometry data in one easy-to-use framework.
Dr Shahram Kordasti, School of Cancer & Pharmaceutical Sciences
He continued: “We want all researchers to benefit from the package, no matter their computational experience, and we want to encourage easy comparison of different experiments within lab groups, as well as externally.”
ImmunoCluster is based in R and involves three core computational stages: (1) data import and quality control; (2) dimensionality reduction and unsupervised clustering; and (3) annotation and differential testing. The framework has been designed to allow scientists with only a basic knowledge of R to perform such analyses, rather than requiring the need for specialist bio-informatic support which can restrict the implementation of such computational analyses for many labs.
A goal of the authors is to disseminate the ImmunoCluster framework to make the wider research community aware of the capability of the package to aid their data analyses.
The ImmunoCluster R package will broaden the accessibility of computational tools for the analysis of high-dimensional cytometry data, facilitating analysis of these complex single-cell datasets.
James Opzoomer (Joint First Author), School of Cancer & Pharmaceutical Sciences
The ImmunoCluster R package will make high-dimensional cytometry data analysis more accessible to a broad range of researchers and support collaborative research projects.
Jessica Timms (Joint First Author), School of Cancer & Pharmaceutical Sciences
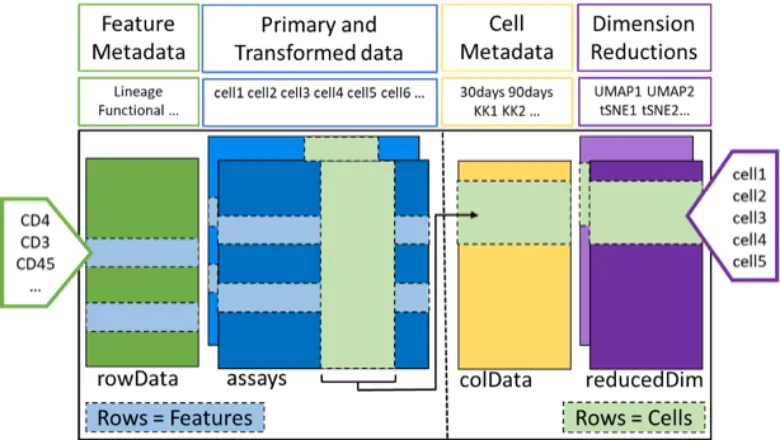