Fluorescence microscopy is one of the most common techniques used in cell biology research. It allows researchers to specifically label structures of interest and observe these within living samples. However, despite the popularity of fluorescence microscopy, there is still very little robust guidance on what makes a ‘good’ image. Obtaining good images is a particular challenge when imaging live cells, as the microscope settings required to produce a visually pleasing image can in fact damage (or even kill!) the sample.
Our group aims to reveal what factors impact the quality of images obtained using fluorescence microscopy, how this affects the accuracy of measurements that can be made from images, and how we can develop image analysis methods to improve the quality of microscopy data.
Our Partners
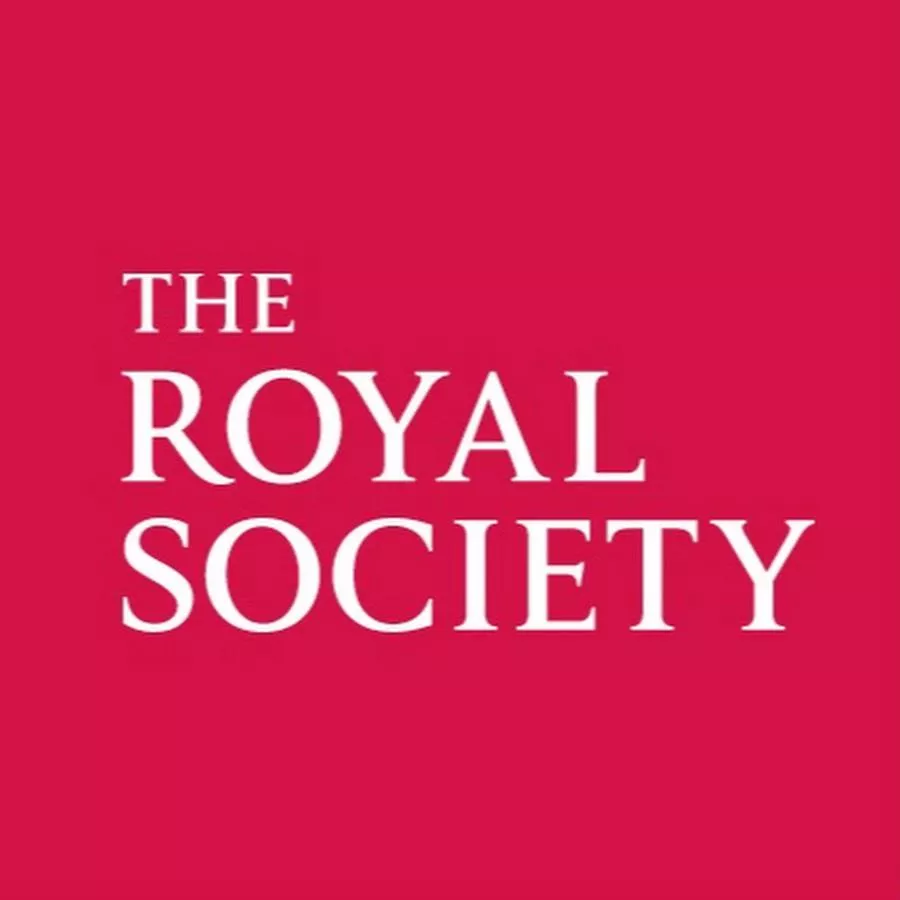
Royal Society