AutoProstate will help radiologists detect prostate cancers earlier, which will save lives. Most men who receive an early diagnosis of prostate cancer will survive their disease for five years or more, compared to only half of men who receive a late-stage diagnosis.
Mr Pritesh Mehta, lead researcher
17 December 2021
Researchers develop AutoProstate to automatically generate prostate cancer diagnostic reports using deep learning
AutoProstate uses deep learning to generate an automatic report to aid radiologists in identifying and diagnosing prostate cancers with greater accuracy
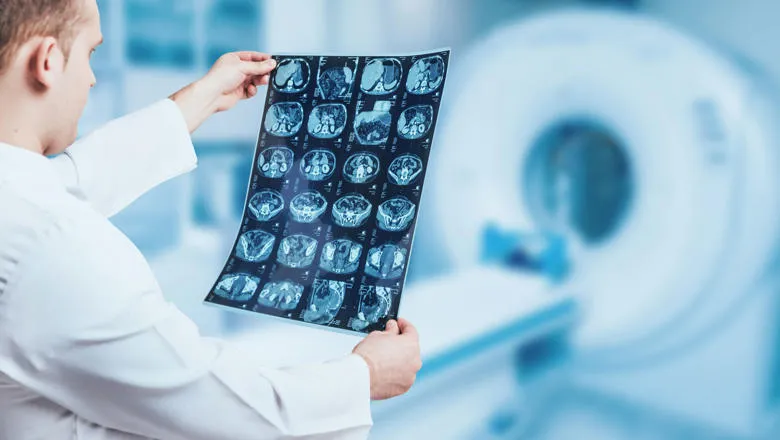
Researchers from the School of Biomedical Engineering & Imaging Sciences have developed a computer-aided prostate cancer diagnosis system, AutoProstate, which uses deep learning to generate an automatic report which will aid radiologists in identifying and diagnosing prostate cancers with greater accuracy. Their findings were published in Cancers.
The current clinical pathway gives rise to missed cancers, which increases mortality risk, and unnecessary biopsies, with side effects that can include bleeding, infection, and difficulty urinating.
Current pressures on the pathway are set to worsen due to rising case incidence and an increasing shortage of specialist radiologists to read prostate MRI.
The researchers behind AutoProstate say artificial intelligence holds the key to improving the diagnostic accuracy of radiologists, as well as alleviating pressures on the pathway.
AutoProstate is a deep learning-powered framework for automatic prostate cancer assessment and reporting.
On how AutoProstate will fit into the clinical pathway, Mr Mehta explained that following multiparametric MRI, AutoProstate will segment the whole prostate, the prostate’s zonal anatomy, and any clinically significant tumours that are present within the prostate, using a patient’s scans.
Subsequently, an automatic diagnostic report will be generated, which will be available to the examining radiologist.
“The automatic report generated by AutoProstate will provide radiologists with additional information at the time of diagnosis, helping to improve diagnostic accuracy, save time, and enhance reporting quality,” Mr Mehta said.
The automatic report is novel in structure and features four main sections: Patient Details, Prostate Size and PSA Density, Clinically Significant Lesion Candidates, and Findings Summary.
“A key benefit of the report is that it automates the calculation of several prostate and lesion-level biomarkers, replacing the crude estimation methods that are currently used,” Mr Mehta said.
“Crucially, all of the biomarkers are easily verifiable as the segmentation outputs of AutoProstate, used to calculate the biomarkers, are displayed in the report in an interactive way.”
In an initial trial of their system, the researchers trained the system using a publicly available dataset released by Radboud University Medical Center, and externally validated the system using data from the PICTURE trial that was run at University College London in 2014.
On evaluation, AutoProstate was shown to perform at the level of a highly experienced radiologist. The strength of our results has provided the necessary evidence for future multicenter external validation and prospective validation. Above all, we hope to show a clinical benefit for less experienced radiologists, who stand to benefit most from the diagnostic information provided by our report.
Mr Pritesh Mehta