"There needs to be a holistic understanding of content, where machine reasoning about image and text is simultaneous. It is not sufficient to rely on the text or the image modality alone for correct interpretation of content – maybe a text on its own isn’t so bad, but if you put it together with a particular image, a highly offensive meaning may emerge."
Professor Helen Yannakoudakis
11 May 2022
Making machines better learners
Written by Juljan Krause
Professor Helen Yannakoudakis from the Department of Informatics is working on the next generation of machine learning models for natural language processing that can learn more effectively and with less training data. Along the way, she is also making existing approaches better from detecting hateful memes to supporting health diagnostics.
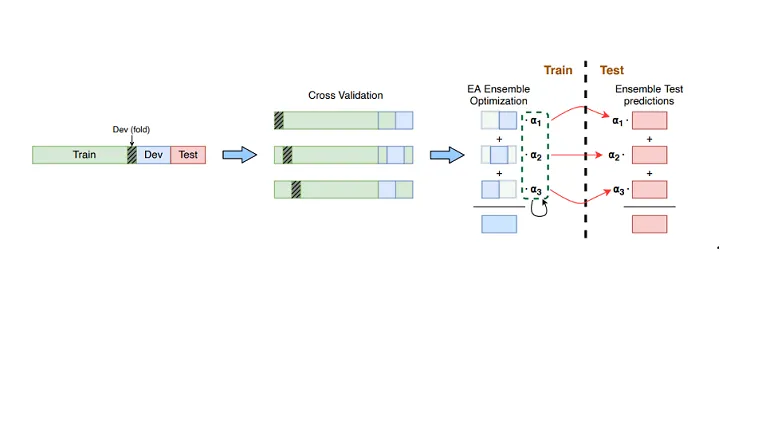
Introduction
We humans are pretty good at learning new things, and we have a smart way of identifying what is in front of our eyes. We don’t need to see hundreds of different tables in order for us to understand that we’re being shown ours when we step into a new restaurant for the first time. And we tend to build on what we already know when we learn something new.
Machines, however, are not quite like that. 'Learning quickly and accumulating knowledge over time pose a particular challenge for machine learning models’, Professor of Computer Science, Helen Yannakoudakis explains. 'At present, machines largely learn by being fed vast amounts of training data: the more examples of a dog the machine has been shown, the more confidently it can identify one in an image it hasn’t seen before. Alas, if the training data isn’t comprehensive enough, machines may misinterpret data.'
Few-shot Lifelong learners, just like us
Recent research on algorithmic bias and algorithmic accountability demonstrates how machines can extend discriminatory practices on the grounds of gender, race or ethnicity, even if only unwittingly so, when training data hadn’t been inclusive. So one way of avoiding such failure is to make machines less reliant on copious amounts of training data when they learn. This means making them smarter in the way they learn over their lifecycle, and that is just what Professor Yannakoudakis has set out to do.
The task is to build machines that are lifelong learners and can adapt quickly from a few examples (aka few-shot learners). ‘For us, this means developing few-shot learners that can continuously learn sequential streams of data quickly, while retaining the knowledge that they have already acquired’, Professor Yannakoudakis continues. Ultimately, the goal is to bring these two strands of research together to build machines that not only learn continuously but can do so with a relatively small amount of data.
Developing this new generation of machines is a complex task that will take some time to take full effect. Notably, however, Helen’s work presents substantial progress on both lifelong learning and few-shot learning in monolingual and cross-lingual settings and has been published in leading venues in the field, such as NeurIPS and ACL, with the most recent one to appear at ACL 2022.
Furthermore, there is plenty of scope to make existing machine learning approaches better. Over the past few years, Professor Yannakoudakis and her team (based at King’s Department of Informatics Human-Centred Computing Research Group) and colleagues have been involved in several key projects that have significantly improved large-scale machine learning models in practice – one of which even earned them a top spot and prize in a global Meta (Facebook) competition.
When a picture is worse than a thousand words: hateful meme detection
Social media is notorious for hate speech and online abuse. Hate on social media is arguably one of the biggest social challenges today – the recent torrent of abuse that England’s football players had to endure for missing their penalty kicks in the finals of the Euro 2021 tournament serves as a stark reminder.
While progress has been made in the automated detection of hateful language, identifying increasingly popular hateful memes remains a massive challenge. ‘People don’t necessarily use swear words, they may use sarcasm, for example, or offensive analogies’, Professor Yannakoudakis clarifies. This is difficult enough for a machine to discern. Images further exacerbate this complexity. ‘There needs to be a holistic understanding of content, where machine reasoning about image and text is simultaneous. It is not sufficient to rely on the text or the image modality alone for correct interpretation of content – maybe a text on its own isn’t so bad, but if you put it together with a particular image, a highly offensive meaning may emerge’, she continues.
Social media giant Meta (Facebook) had set out to tackle this problem, so Professor Yannakoudakis formed a team and together with colleagues from the University of Amsterdam responded to its ‘Hateful Memes Challenge’ in 2020. Having built a novel framework that makes for better training of algorithms and swifter detection of multimodal hate memes in large datasets, the team finished in the top five among over 3,000 participants. Their success didn’t just earn the team a cash prize but also the opportunity to publish and present their work at the prestigious Conference on Neural Information Processing Systems (NeurIPS) in 2020, while Helen was subsequently invited for a Spotlight interview by DrivenData (to appear).
Health diagnostics and emotion detection
Being at the receiving end of online abuse can have a severe impact on mental health. In many instances, a sooner and better diagnosis could make for faster intervention. ‘We’re talking about an approach that involves the detection of mental ill-health based on an individual’s use of language’, Professor Yannakoudakis outlines a further research topic she is pursuing at Kami (CUSP London). She is quick to point out that the task is not to replace the work of health care professionals. ‘A diagnosis is a rather strong word – machines are not doctors, and this technology is not to replace doctors’, she clarifies. ‘But maybe we can help detect early signs of depression, self-harm or suicide risk, and facilitate and support diagnosis of mental ill-health through the use of AI technology’, she continues.
Mental health is inextricably linked to one’s emotional state. In a recent paper, Helen and colleagues have built a joint model of detecting emotional patterns and abusive language simultaneously. Experimental results from a multi-task learning environment suggest that incorporating affective characteristics in this way makes for much better automated abuse detection on online platforms. Pairing emotion detection and identifying hate speech is a promising approach to making machine learning models better at tackling this massive social problem and support mental health diagnostics further down the line.
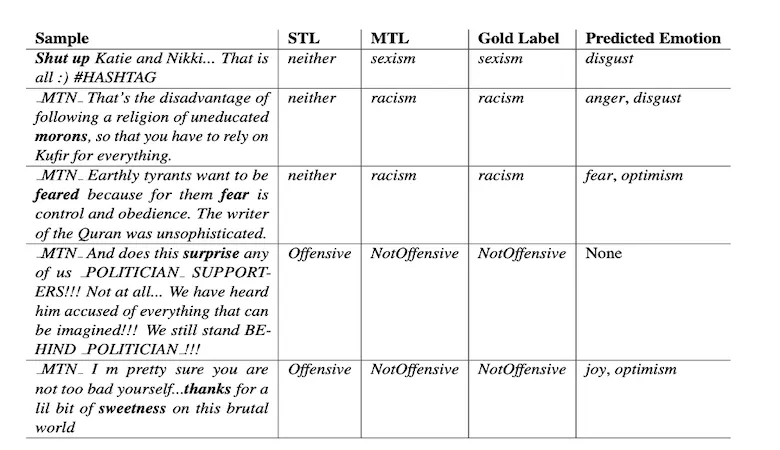
Looking ahead: the next steps
Throughout her varied work across machine learning and natural language processing, Professor Yannakoudakis lauds the collaborative and inspiring work environment at the Department of Informatics. ‘The intersecting structure of networks, groups and hubs in Informatics, as well as CUSP London, guest speakers and reading groups at the department, among others, bring together thematic areas of research and offer a collaborative and stimulating environment where inter- and multi-disciplinary research can thrive’, she adds.
Moving forward, Professor Yannakoudakis has several new projects in the pipeline. There is her continuous collaboration with Cambridge University that supports students in their essay writing. The tool they have built automatically assesses written work in seconds. Several interdisciplinary projects with other Departments and colleagues at King’s are also well underway, notably with Dr Rita Borgo on explainability and visualisation of dialogue systems, and Dr Elisa Cavatorta from the Department of Political Economy on investigating language in conflict situations. Showing no signs of slowing down, Professor Yannakoudakis is set to continue paving the way for the next generation of machine learning and natural language processing models.