But with multimorbidity, events (i.e. the onset of long-term conditions) overlap, rather than happening one after the other; having diabetes does not imply recovery from high blood pressure or high cholesterol. The accumulation of long-term conditions, rather than a simple transition from one state to another, requires innovative strategies for analysing multimorbidity.
Dr Marc Delord
17 February 2025
Machine learning uncovers patterns of multimorbidity associated with stroke
Researchers at King’s College London have developed and used a machine learning approach to map patterns of multimorbidity associated with stroke over time using data from primary care electronic health records.
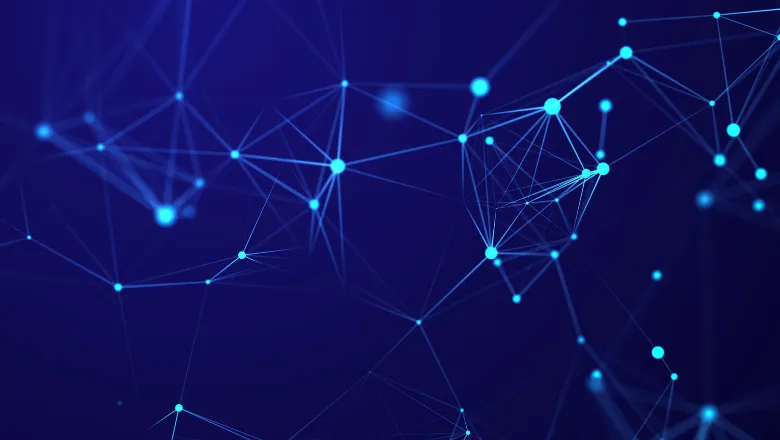
Multimorbidity is defined as the presence of two or more long-term health conditions in an individual at the same time, and its prevalence increases with age. While routinely collected healthcare data, including dated records of common long-term conditions leading to multimorbidity, are becoming increasingly available, there is a lack of innovative methods to analyse this information and ultimately improve the prevention and treatment of multimorbidity.
Two studies, published in BMC Medical Research Methodology and BMC Primary Care, propose and apply a newly developed machine learning approach to identify the most common health trajectories – or sequences of long-term conditions (multimorbidity) – using electronic health records. This method, applied to patients who have experienced a stroke, provides a novel framework for studying and gaining deeper insights into multimorbidity across the life course.
The approach
“Effectively analysing multimorbidity is challenging” says Dr Marc Delord, Research Fellow in epidemiology at King’s and lead author of the papers. “Here, we have adapted an ‘unsupervised learning’ approach developed in the setting of the social sciences and genomics, where a sequence of events (or a sequence of the genome) will tend to find its match among a wider population until groups or ‘clusters’ of the main type of sequence in the dataset emerge.”
“For instance, a population that has completed a short curriculum at university will tend to get a first job earlier in a life course than a population that has completed a longer curriculum. Consequently, matching algorithms will tend to separate individuals in clusters based on the dated sequences of events ‘university to first job’.”
Helping to understand the complexities of multimorbidity
In the study published in BMC Primary Care, the team used its newly developed machine learning algorithm – named Multiple State Clustering Analysis (MSCA) – to analyse primary care electronic health records linked to up to 30 long-term health conditions, including conditions that are common stroke risk factors such as high blood pressure and diabetes, in 9,847 patients who had experienced a stroke. The records were collected on patients who were over 18 years of age and registered in 41 general practices in south London between April 2005 and April 2021.
MSCA is an unsupervised approach, which means that the algorithm can recognise patterns within a dataset without being given specific instructions.
The analysis revealed eight clusters of patients from the electronic health records. Each cluster had a distinct pattern of multimorbidity, levels of medication, socio-demographic profiles, and stroke risk factors, and showed specific trends in age and ethnicity.
A core of three clusters were associated with conventional stroke risk factors. Smaller clusters exhibited less-common combinations of long-term conditions associated with stroke, including mental health conditions, asthma, osteoarthritis and sickle cell anaemia. More complex patient profiles combining mental health conditions, infectious diseases and substance dependency also emerged.
The method was able to identify specific patterns of multimorbidity, including repeated patterns displayed in various subpopulations. For example, the sequence of high blood pressure to stroke was spotted at different ages and socio-demographic contexts in three different clusters.
Our findings highlight the main groups of health characteristics observed in the general population among individuals who have experienced a stroke. They also provide a valuable foundation for future research aimed at exploring specific patterns that may play a causative role in stroke onset.
Dr Delord
The proposed method offers a novel approach to studying and understanding the complexities of multimorbidity, with the identified patterns also having the potential to inform future care and prevention strategies across diverse patient populations.