This method develops a clinical marker and automates its extraction which would otherwise be prohibitively time-consuming in clinical practice. The validation on an independent clinical cohort with MRI data acquired at a different field strength shows that it can be a valuable clinical tool.
Dr Maximilian Pietsch, Research Associate, School of Biomedical Engineering & Imaging Sciences
09 August 2021
Health of placenta can be predicted in 30 second MRI scan, say researchers
The scan together with the automatic pipeline can easily be included in any fetal MRI examination allowing new information for better treatment & information

Researchers from the School of Biomedical Engineering & Imaging Sciences propose a machine learning method that predicts the health of the placenta from a thirty second MRI scan. The algorithm models the normal distribution of placental tissue properties which can be used to screen for deviations from normal placental ageing and signs of pregnancy complications.
The researchers use this method to detect pre-eclampsia, a condition that affects 4 to 7 percent of pregnancies with significant mortality and morbidity for both mother and child.
The study, published in Medical Image Analysis was validated in two cohorts on two scanners and with histopathology data.
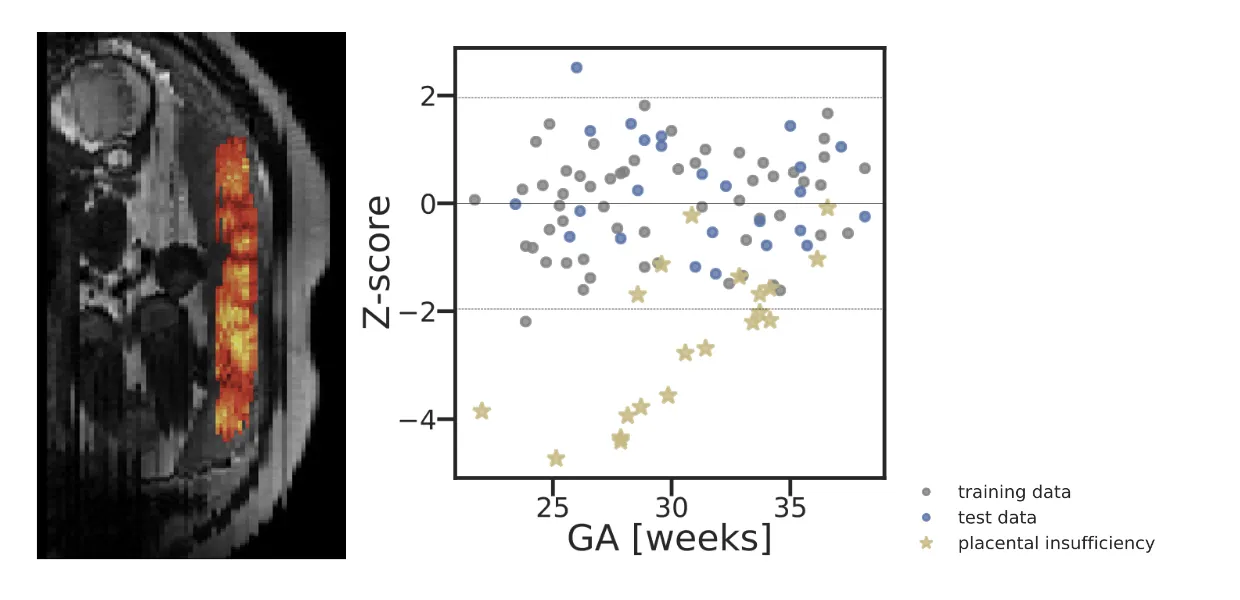
The thirty second scan together with the automatic pipeline can easily be included in any fetal MRI examination and allows obtaining additional, previously not available information leading to better treatment and information.
Lead researcher Dr Maximilian Pietsch said detecting pre-eclampsia is essential to achieve optimal monitoring and thus the best possible outcome. The method is most sensitive early in the second half of pregnancy which is also the typical time of onset of pre-eclampsia. This allows early detection and monitoring of this complication.
The machine learning pipeline automates manual segmentation of the placenta, which can take up to one hour per case, and uses data-driven models to define normal intervals of tissue properties within the placenta. This new technique is fast and removes any laborious steps. At the same time, it uses human-inspectable and interpretable intermediate representations of the data, keeping clinicians in the loop and allowing optional corrections and alleviating issues related to black-box algorithms for clinical decision making.
Also, incorporating the uncertainty associated with the data in the model improves the method’s ability to assign lower health scores to high-risk placentas (AUC ROC) from 69 percent to 95 percent.
This is a very exciting step forward towards using the novel MRI techniques in clinical practice - enabled by the interdisciplinary setup here at St Thomas' Hospital and the close collaboration between obstetricians, physicists, radiologists, midwives, engineers and pathologists!
Dr Jana Hutter, Research Fellow in the Department of Biomedical Engineering, School of Biomedical Engineering & Imaging Sciences