We need to dissociate human language from intelligence just as we were told by Alan Turing. Our research shows that advanced chatbots can fail when it comes to fundamental abstraction and predictive capabilities. SuperARC is a step towards an objective, universal test—one that can spot whether an AI system is genuinely moving the needle on general or superintelligent behaviour, rather than just emulating human-like behaviour without meaning the words.
Dr Hector Zenil, senior author, Associate Professor/Senior Lecturer at King’s College London and Founder of Oxford Immune Algorithmics, a spinout leading the application of Superintelligence to healthcare
22 April 2025
New study introduces a test for artificial superintelligence
Researchers propose a new benchmark that uses advanced compression and a specialised type of probability to find the most likely explanations for Artificial Superintelligence (ASI) without relying on human-focused assumptions, as other tests do.
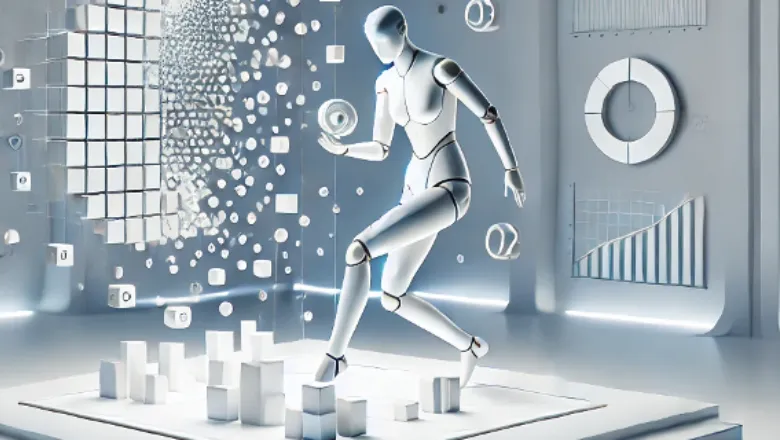
A research team led by Dr Hector Zenil, Senior Lecturer and Associate Professor at the School of Biomedical Engineering & Imaging Sciences, King's College London and Founder of Oxford Immune Algorithmics, has published a paper that puts forward an innovative benchmark—referred to as the SuperARC framework. Inspired by the original ARC challenge, this new test is designed to evaluate whether current and future AI systems possess the foundational characteristics required for artificial superintelligence (ASI).
The findings show that current leading large language models (LLMs) such as ChatGTP, DeepSeek or Qwen are still far from AGI or ASI and often circle around the same intelligence levels.
SuperARC defines intelligence in terms of recursive compression repeatedly condensing information to reveal deeper patterns not apparent to tools such as Large Language Model chatbots (LLMs). The test employs a type of specialised probability, drawing upon the equivalence between compressibility and predictability established in the theory of randomness. The paper proves mathematically the equivalence between compression and prediction and exploits it to show how model abstraction and planning in the context of AI are formally two sides of the same coin.
The authors argue that intelligence is best measured by the ability to produce approximations to short computable hypotheses—one that can not only reconstruct but also predict data by running code in parallel to simulate many future states and pick the one that is closer to the observation at any given time. This perspective moves away from conventional, human-centric IQ-style tests, aiming for a more fundamental and agnostic measure of natural and artificial higher cognitive ability not based on human-centric single answers.
The test also makes it more difficult for current AI systems and frontier models to cheat which is something current systems are implicitly or explicitly doing when training on the very answers they are tested on.
Multiple leading LLMs (including GPT variants, DeepSeek, Qwen, Grok, Claude, Gemini, Meta, and others) were tested on tasks requiring model abstraction, inverse problem-solving, and short-sequence prediction and generation. Despite their linguistic prowess, these systems generally failed to model and generalise beyond trivial “print” solutions, that is, simply answering back with the original question. The study thus raises questions about LLM convergence on higher-level reasoning or merely amplifying pattern matching from ever larger sources of big data.
Results indicate no clear breakthroughs towards AGI or ASI, particularly for tasks requiring true model inference and robust planning. Notably, newer versions of the same LLMs occasionally performed worse than their predecessors, suggesting no consistent upward trajectory in less-human centric intelligence metrics. This also suggests LLM teams are focusing on optimising for ever changing human-centric tests for AI in their attempt to appear more intelligent rather than being so.
This study fits with what I’ve been arguing for years—Large Language Models, despite their hype, are not moving towards real intelligence; they continue to struggle with abstraction, reasoning, and planning. These results suggests that LLMs are not converging to anything resembling general intelligence, but instead remain brittle, erratic, and deeply dependent on the specific data they memorise —suggesting we need a new approach.
Prof. Gary Marcus, Professor of Psychology and Neural Science (Emeritus), NYU, and Founder and Executive Chairman of Robust AI
The authors of this study propose that future AI progress hinges on integrating symbolic inference with machine learning, arguing that “pure memorisation” approaches fall short of genuine comprehension. A shift to neurosymbolic models may be required to bridge the gap between advanced pattern recognition and true algorithmic inference.
The paper makes compelling arguments about the limitations of current LLMs, demonstrating that despite their impressive language capabilities, they fall short on more fundamental measures of intelligence according to the SuperARC metrics. Their proposed hybrid neurosymbolic approach, Block Decomposition Methods (BDM), clearly warrants further exploration.
Prof. Mark Bishop, Scientific Advisor to FACT360, and Professor of Cognitive Computing (Emeritus), Goldsmiths, University of London
Dr Zenil believes that this more reliable form of superintelligence—one not solely reliant on LLMs—will be key to addressing major human challenges such as disease, and will transform healthcare.
Read the full paper here.
Attend Dr. Zenil’s talk at the King's Festival of Artificial Intelligence on How Artificial Super Intelligence Will Solve Human Disease.