This has been one of the most inspiring research events I've attended in many years!"
Elena Simperl, Professor of Computer Science
25 August 2023
King's hackathon explores solutions to making generative AI more trustworthy and accurate
The hackathon brought together researchers and practitioners with an interest in using the latest advances in pre-trained language models (PLMs) and generative AI.
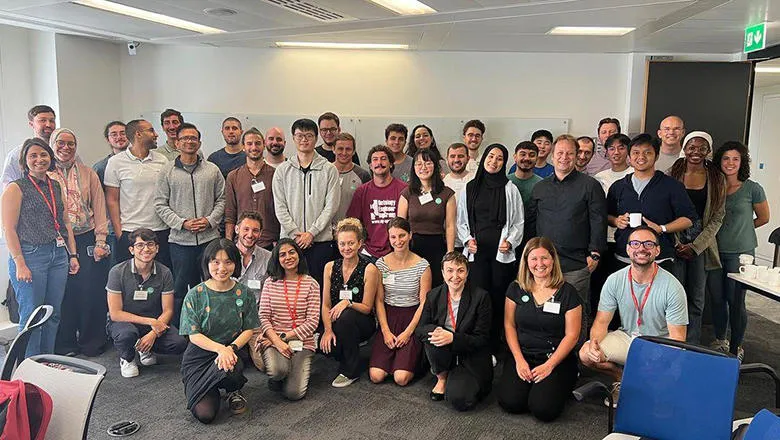
A hackathon organised by the Department of Informatics this month explored how generative AI technologies such as ChatGPT could help individuals and organisations create, access, and share trustworthy knowledge and information.
The event which spanned over four days saw over 40 PhD students and researchers from King’s and universities from mainland Europe hack solutions to improving trustworthy generative AI at Bush House, King’s College London.
Generative AI offers many opportunities but has come under criticism due to its capacity to generate factually wrong or fake information, which it presents confidently to users as the truth. By contrast, knowledge graphs and knowledge bases are curated and assessed by real people to help web search engines, platforms such as Wikipedia or intelligent assistants like Alexa and Siri deliver actual facts alongside their provenance.
Professor Elena Simperl and Dr Albert Meroño from Informatics partnered with four other European universities in Bologna, Amsterdam, Madrid, and Vienna to deliver a hackathon exploring how to use generative AI tools to create knowledge graphs and ontologies during the hackathon. It was supported by technology companies such as Data Language and metaphacts, as well as several large research programmes and organisations, including Polifonia, MuseIT, ENEXA, and the Alan Turing Institute.
The hackathon was organised as a collaborative, interdisciplinary sprint-style research activity in which participants worked in teams to prototype new ideas, methods, tools, and evaluation frameworks around the use of large language models to produce, access, and share knowledge that people can trust. The gathering initiated an interdisciplinary community of interest and practice in deploying advanced AI capabilities in support of engineering better knowledge graphs for trustworthy, human-centric information services, from search and question answering to recommendations and fact checking.
Working in teams of six for four days, participants were allocated to a series of curated topics, each supervised by a mentor based on their research background, which included:
- Using Pre-trained Language Models (PLMs) or Large Language Models (LLMs) to support specialised knowledge engineering tasks such as generating competency questions, evaluating knowledge graphs from an ontological perspective, aligning across knowledge graphs and their underlying schemas, extracting rich knowledge structures beyond simple entities and relations
- Understanding the benefits and limitations of conversational affordances, i.e. chatbots like ChatGPT, as an alternative to established knowledge graphs with respect to usability, task performance, and the ability to gain and maintain user trust.
- Methods to ensure human-in-the-loop knowledge base construction - i.e. knowledge with human input - is transparent, accountable, fair, and compliant with emerging AI laws and regulations.
We aim to incorporate more diverse data sources to ensure comprehensive and unbiased knowledge extraction."
Anthony Hughes from Data Language
Elena Simperl, Professor of Computer Science at the Department of Informatics and one of the organisers of the hackathon, said:
“This has been one of the most inspiring research events I've attended in many years! In four days we learned about the different aspects of knowledge engineering that LLMs could support and I can see many interesting human-LLM challenges emerging which are unique to our field”.
Anthony Hughes from Data Language, who worked in one of the teams working using large language models to extract knowledge, also shared his insights:
“Overall, the Group A team gave us insights to a range of potential business capabilities regarding both knowledge graphs and language models.”
He also mentioned their next steps, which are part of the further impact of this hackathon:
“Building on our initial results with knowledge base construction using Pre-trained Language Models (PLMs), the team's next steps involve refining and expanding the methodologies. We aim to incorporate more diverse data sources to ensure comprehensive and unbiased knowledge extraction. Further, we'll delve deeper into advanced fine-tuning techniques, enhancing our model's capability to discern nuanced relations and handle ambiguities.”
The hackathon will produce a report outlining their findings and recommendations to inform best practice in this area.
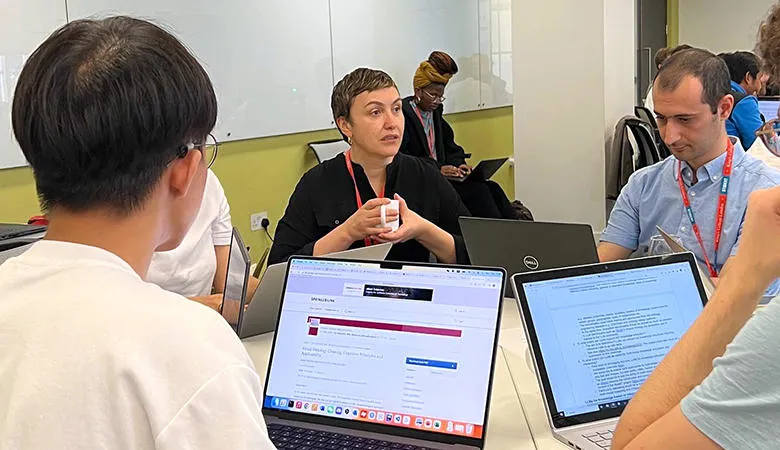