In some ways, young people with child protection plans have worse outcomes than looked-after children. Their absence from major datasets makes it harder to see this, and might mean that they’re ignored by well-intentioned policies.
Michael Sanders and Louise Reid
21 August 2019
How data and machine learning can help strengthen communities
Michael Sanders and Louise Reid
MICHAEL SANDERS AND LOUISE REID: New frontiers are opening up in the way we use big data and machine learning which could be used to help our communities thrive
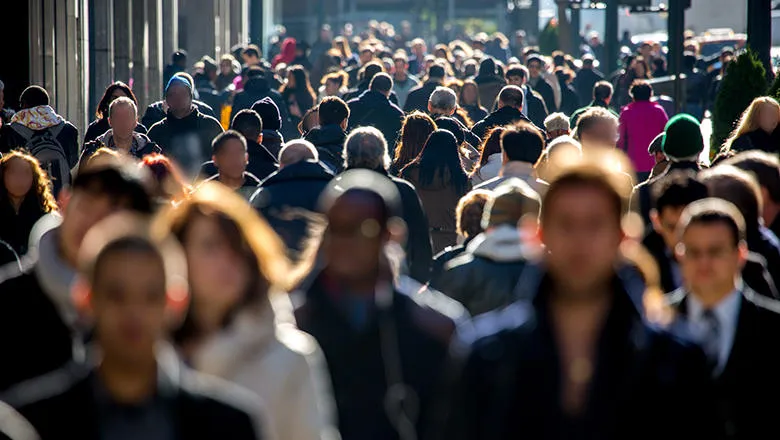
This piece was originally written for the Policy Institute's new Policy Review publication, which features essay contributions on a range of different issues by the institute’s researchers and Visiting Faculty.
Read the full collection of essays.
Armies of researchers and data scientists at universities and in the public and private sectors are trying to make use of advances in the availability of data and in computing power. There are interesting frontiers in these areas, and they could be used to help our communities thrive. There are three main areas that we see as key to bringing about this change: machine learning and predictive analytics, better descriptive data, and better understanding relationships.
Predictive analytics
One of the major uses of machine learning is to predict the future based on past events. The world is an ever-changing place, so this can never be perfect, but a computer can make inferences based on everything that’s happened before, and what tended to happen next. Unlike with humans, emotions, boredom or inherent biases don’t play a part – although the machine will tend to learn from any bias that already exists, like implicit or explicit racism, sexism, or ageism. We need to make sure we understand – and are checking for – bias in our models, but where these biases are either small or can be corrected for, there is a lot of potential to direct targeted government services. This targeting would allow government to use money more effectively by deploying it where it is likely to do the most good, rather than spreading it more thinly across a wider number of cases, many of which may be lower-risk. Bringing additional datasets into the mix would let us reduce or better identify bias, improve accuracy, and build a more holistic picture of the problem we’re aiming to tackle.
Who gets measured, gets helped
It’s a truism of government accountability that “what gets measured, gets done”, but the more data we have and the more it is used to target services, the more we will see a new paradigm emerge of “who gets measured, gets helped”. We see this in a number of ways, for types of characteristics which, for one reason or another, tend to appear less often in large datasets that shape policy. In children’s social care, for example, government datasets will usually have an indicator for whether or not a child has been in foster care, either at the moment or in the past. So we can see, for example, how well those young people do at school, and how likely they are to go to university. We see it in later life as well, where research tells us that 20% of rough sleepers are care leavers, a fact which has recently prompted the British government to invest £5 million in reducing homelessness among this group.
Young people who have, or have had, child protection plans – the next rung down the statutory child protection ladder – are found much less frequently in the datasets that researchers use. This means that there are far fewer studies looking at these young people and their outcomes – even though they still have very difficult lives – than young people who have been taken into care. Where research has been done, for example in the United States, and by the Rees centre at the University of Oxford, we see that in some ways, young people with child protection plans have worse outcomes than looked-after children. Their absence from major datasets makes it harder to see this, and might mean that they’re ignored by well-intentioned policies.
The same is true for the LGBT+ community. Data on people’s gender or sexuality have to date not been collected in the UK’s decennial census – 2021 will be the first time it asks people for this information. But the Office for National Statistics is concerned that there will be a high level of non-response or inaccurate response. The majority of datasets used by government and by researchers are silent on LGBT+ issues, and so studies can’t reflect the lived experiences of people. We know from research on lower-income students and people of colour that a sense of social distance can alienate them from education and lead to worse outcomes – and these findings have led to interventions to close the gap – but for LGBT+ students, who are largely invisible in our data, we miss them entirely. Better-quality descriptive data, and a more systematic approach to asking questions relating to factors that might be relevant, is going to be essential if data are going to be used to improve outcomes for everyone in society.
Better understanding relationships
Human relationships are complex and multifaceted, and as such are pretty hard to understand, even for other human beings, let alone computers. This may be why the most prominent use of matching algorithms in human relationships have been limited to relationships which are, shall we say, brief.
More computing power, and our ever-growing set of connections, offers the prospect that we can better understand how information flows through relationships, and the kinds of relationships that are likely to be successful. Some of this is already being put into use by some of our former colleagues, who have developed an app which helps bridge the social divide between groups and enables people to form more – and more inspiring – friendships.
This is not just an exercise in social mixing, however. There is a growing consensus that our tendency to share information with one another, and to rely on each other for social signals about what is right and true, has been hijacked by organisations looking to manipulate us into buying X product or voting for Y cause. Early analysis of data relating to “fake news” on Facebook showed a disturbing prevalence of such news even before the more recent scandals brought it to public attention, and that attempts to curb the sharing of such content were only moderately effective. 10 years later, policymakers around the world need not to leave this kind of analysis to those who would do us ill, but must use their power – both computing and legislative – to better understand it and build tools to combat its abuse.
Conversation needed
We’ve shown here three ways that data can be used. Each carries risks – such as targeting the wrong people due to bias, or of encouraging (or at least failing to prevent) nefarious actions online. But a revolution in the use of data isn’t coming – it has already made substantial strides. If they are to keep abreast of this rapidly changing digital world, policymakers have two responsibilities: to get to grips with data and its uses while trying to ensure it is used for good, and to create a loud, boisterous public debate on these issues – without which this research, and its use in policy, cannot have democratic accountability.
Dr Michael Sanders is a Reader in Public Polic at the Policy Institute, King’s College London, and Executive Director of the What Works Centre for Children’s Social Care.
Louise Reid is Head of Programmes and Interim Head of Research at the What Works Centre for Children’s Social Care.